Effectiveness and Adherence to Physical Activity and Physical Exercise mHealth Interventions: A Systematic Review
Mireya Fernández-Chimeno
Violeta Moize Arcone
José Antonio Sánchez-Fuentes
Eva Aurin Pardo
Carme Carrion
*Corresponding author: Toni Caparrós toni.caparros@gencat.cat
Cite this article
Caparrós Pons, T., Fernández-Chimeno, M., Moize Arcone, V., Sánchez Fuentes, J. A., Aurin Pardo, E. & Carrion, C. (2023). Effectiveness and Adherence to Physical Activity and Physical Exercise mHealth Interventions: A Systematic Review. Apunts Educación Física y Deportes, 151, 1-16. https://doi.org/10.5672/apunts.2014-0983.es.(2023/1).151.01
Abstract
The inclusion of physical activity and physical exercise prescription in the field of mHealth offers a new field of research in an increasingly digitized society. In this context, it is necessary to assess the applicability, reliability and adequacy of the control variables and assessment, taking into account the criteria of individualization and specificity of the pathology. This systematic review was aimed to study the adherence and effectiveness of physical activity or physical exercise mHealth programs depending on its variables, communication channels and technological resources for randomized clinical trials performed from 2011 to 2021. A literature research was carried out using the Pubmed, Science Direct, Scopus, Web of Science and Google Scholar platforms following the Preferred Reporting Items for Systematic Reviews and Meta-Analyses (PRISMA) guidelines. Out of 865 initial results, 13 clinical studies were included in the analysis, related to cardiac (n = 4), oncological (n = 3), pulmonary n = 1), Parkinson’s (n = 1) diseases, as well as the promotion of PA in sedentary people (n = 2), general population (n = 1) and pregnant women (n = 1). In relation to the effectiveness of the intervention, 9 of the final results (70 %) showed differences of improvement in the experimental group. Workload is usually quantified with non-specific variables, daily steps and the minutes of physical activity or exercise per day being the most with common ones. The most used technological resources were specific applications and the FitBit® wristband. Main communication channels were SMS and the social network Facebook. However, there were no interventions specifically designed to meet technological capabilities of their target population. mHealth interventions had a greater effectiveness and adherence to prescription programs than in those prescribed in person or without technological support.
Introduction
Regular physical activity (PA) offers significant health benefits and mitigates health risks. It is defined by the World Health Organization (WHO) as a key protective factor for the prevention and management of noncommunicable diseases, for its benefits for mental health and delaying the onset of dementia, and for its contribution to the maintenance of healthy weight and general well-being (Bull et al., 2020). The American College of Sports Medicine defines PA as “the body movement that is produced by the contraction of skeletal muscles and that increases energy expenditure”. Physical exercise (PE), on the other hand, refers “to planned, structured, and repetitive movement to improve or maintain one or more components of physical fitness”. PE and the accumulation of PA improve physical fitness, allowing to assume a state of well-being with a low risk of premature health problems, and the energy to participate regularly in a variety of physical activities (Chodzko-Zajko et al., 2009). In that context, PA and PE prescription and its concurrent inclusion in the field of mobile health or mHealth offer a new field of research in an increasingly digitized society (Watson, 2020).
mHealth, as a component of eHealth, is a medical and public health practice supported by mobile and wireless devices, involving the use of mobile phone functionalities and its applications (WHO, 2015). mHealth is presented as a technological advance that could be useful for the promotion of healthy PA and PE, both in industrialized or rural areas (Griffin et al., 2020). mHealth could be a new solution for the management, assessment and control of PA or PE, taking into account the use of mobile phones in today’s society as well as the emergence of 5G (Silva et al., 2015). It is accessible everywhere and a person does not necessarily need a specific time and place to start exercising. One can also think that it does not need to be attended by a PA and PE professional (Sohaib Aslam et al., 2020), although this current perception could be counterproductive, both for the health of the person who exercises without concrete or specific guidelines in their sports practice and in the interpretation of the PE prescription for health. In this sense, it would be necessary to assess one of the differential effects of sports practice and its adherence, such as socialization (Short et al., 2018). mHealth interventions should be presented as facilitators for the practice of healthy PA and PE, but under mechanisms and control criteria which are individualized to each user or patient and are specifically targeted for each specific group of people suffering from a specific pathology (Paglialonga et al., 2018). However, it is necessary to assess the applicability, reliability and adequacy of the control variables for volume and intensity of PE, taking into account criteria of individualization and specificity of the pathology (Duscha et al., 2018a), as well as the development of technological resources and the communication channel to every specific population group need (Sohaib Aslam et al., 2020).
In that context, exercise prescription is defined by two main workload parameters: external load (EL) and internal load (IL). Both are independent parameters used to assess the effect of exercise, and their control is integrated and ruled by training theory (Foster et al., 2017). A certain EL will generate different physiological and psychological responses in every person; this response is IL (Soligard et al., 2016). Time, steps, distance, accelerometry, sets, repetitions, etc., are variables applicable to the quantification of EL (Mujika, 2017). IL has been useful to guide the training process or control fatigue (Soligard et al., 2016) . Objective variables to measure IL (apart from all physiological parameters analyzed form blood test) are Heart Rate (HR), Heart Rate Variability (HRV) (Capdevila et al., 2008), or those obtained from blood test like myosins or cortisol. A subjective tool in terms of IL is the Rate of Perceived Exertion (RPE) or Borg scale (Muyor, 2013), which is defined as not invasive and ecological (Moreno Sánchez et al., 2013).
EL and IL are related, the first being defined as the external physical stimulus applied to the athlete during exercise or training (Soligard et al., 2016). Individual assessment of the relationships between the two loads offers specific information of each person as a specific tool for controlling its own adaptation processes and recovery (Martín-Guillaumes et al., 2018). With these variables, sports scientists or Physical Education graduates have the possibility of adjusting load according to individual responses (Foster et al., 2017). However, the analysis of these variables during the prescription process would allow to individually design exercises, and to better adapt the workloads to the required health objectives, individual needs, and pathology requirements (Zenko & Ekkekakis, 2015).
This systematic review aims to study the adherence and effectiveness of physical exercise and physical activity mHealth programs depending on its workload variables, communication channels and technological resources.
Methods
This systematic review was registered in PROSPERO (registration number: CRD42021270081) and was reported following the Preferred Reporting Items for Systematic Reviews and Meta-Analyses (PRISMA) guidelines (Page et al., 2021). Due to the methodological and statistical heterogeneity of the studies included, a descriptive approach has been adopted in the research synthesis (Rethlefsen et al., 2021).
Eligibility criteria
The inclusion criteria were randomized clinical trials performed from 2011 to 2021 that assessed effectiveness of and adherence to mHealth programs whose main outcome was to increase physical activity (PA) or physical exercise (PE). All interventions were prior to COVID-19. Interventions mainly based on changes in dietary patterns, mixed virtual and face-to-face interventions, non-controlled trials, letters to the editor, conference abstracts, books, and reviews were excluded. Methodological quality was rated depending on their risk of bias according to the Scottish Intercollegiate Guidelines Network Group (SIGN) grading system, as high (minimal risk of bias), good (moderate risk of bias) and fair (high risk of bias) methodological quality (Harbour & Miller, 2001). No studies were excluded on the basis of methodological quality.
Information sources
A systematic search was conducted in the following databases: PubMed, Science Direct, Scopus, Web of Science and Google Scholar. The survey period included all articles published from January 1, 2011 to August 30, 2021.
Search strategy
The search strategy included both controlled-vocabulary and free-text terms. The terms used were “physical activity”, “physical exercise”, “mHealth” and “eHealth” (see Table 1).

Table 1
Search strategy for “Physical activity and physical exercise mHealth interventions”.
Study selection and data collection process
All identified references were imported into Mendeley v1.19.8 (Elsevier) and duplicates were eliminated. A total of 3 researchers undertook the review process, which was conducted in 3 stages. As a first step, the titles, abstracts, and keywords of the relevant studies were screened by the authors. In the second step, the full-text articles were screened, while in the third step the reference lists of the included articles and of review articles on PA and PE prescription in mHealth were searched for additional articles. None of these were included. Any disagreement was discussed until consensus was reached.
A protocol was developed for data extraction from the articles. Information was extracted related to the article (author, year of publication), participants (number, gender, mean age), objective, pathology, intervention (description of intervention, duration, physical activity or exercise prescription, communication channel), workload variables, mHealth tools, results (conditioning, quality of life, adherence), and conclusions.
Results
Selection of the studies
A total of 403 potentially relevant publications were identified as eligible. After screening the titles and abstracts, 40 (9.9 %) were accepted for full-text review. Out of these 40, 27 (67 %) were excluded for not meeting the inclusion criteria: not presenting variables related to PA or PE, not describing the prescribed PE protocol (n = 3), prescription of PE with mixed care (face-to-face and virtual) (n = 5), not using a control group (n = 2) or using a PA protocol that did not meet the aim of the review (n = 16). After peer review, 13 articles were included in this non-quantitative review (see Figure 1; see Table 2).
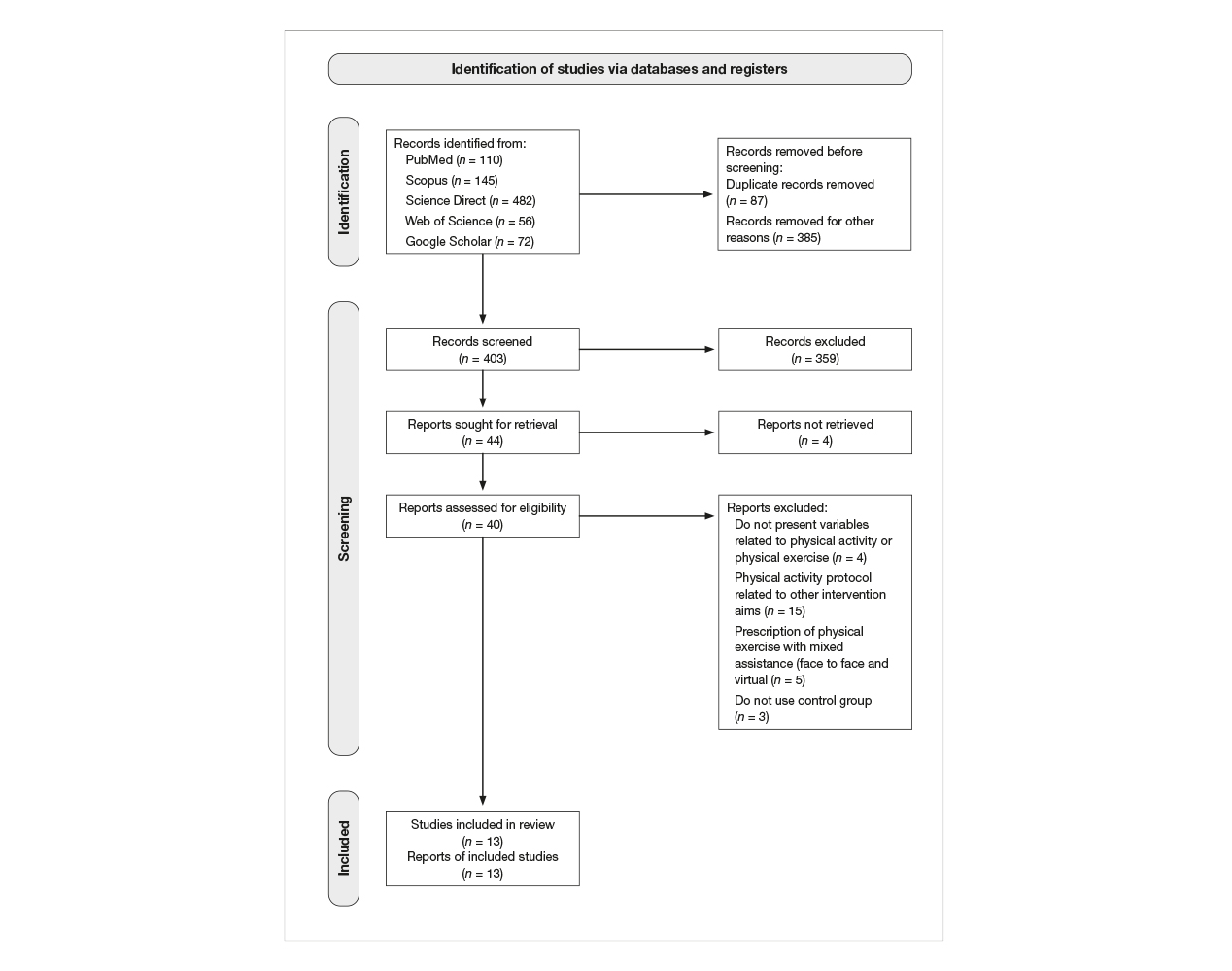

The main characteristics of the 13 studies included are set out in Table 3. Studies appear in alphabetical order of the first author within chronological years. All the selected studies focused on mHealth interventions to identify their effectiveness, PA load variables, technology, and communication channels. All studies were clinical trials. All studies were compared pre and post outcomes to analyze the effectiveness of the intervention, but just 4 of them (30 %) followed the CONSORT criteria (Hopewell et al., 2008). According to SIGN criteria, the majority of the studies were of low quality (n = 12), with only one of good quality. A fair quality rating most often resulted from small sample size, inadequate length of study, or possible selection and information bias.

The studies presented have a high clinical heterogeneity, according to the number of participants in the trials, the type of pathologies and the duration of the intervention (Page et al., 2021). The number of participants ranged from 15 to 2,783 and just over half of the studies (n = 7) included less than 100 people. Most studies had a majority of adult participants (n = 10); in 4, the participants were adolescents or young adults, and in 2 they were women. Out of the 13 clinical studies included in the analysis, 4 were related to cardiac illness (Duscha et al., 2018a; Duscha et al., 2018b; Klausen et al., 2016; Vasankari et al., 2019), 3 to cancer (Mendoza et al., 2017; Quiñonez et al., 2016; Uhm et al., 2017), 1 to pulmonary disease (Vorrink et al., 2016b), 1 to Parkinson’s disease (Ellis et al., 2019), and 4 to the promotion of PA in sedentary people (Hart et al., 2020; Shcherbina et al., 2019), general population (Martin et al., 2015), and pregnant women (Choi et al., 2016).
The studies were conducted over periods of a week (n = 2), four weeks (n = 1), 10 weeks (n = 1), 12 weeks (n = 4) 90 days (n = 1), six months (n = 1), or a year (n = 3). The countries where the studies were carried out were the United States (n = 10), the Netherlands (n = 2), Finland (n = 1), and Germany (n = 1).
Effectiveness of intervention
With regard to the effectiveness of the intervention in PA-related terms, the final results in 9 cases (70 %) showed differences in improvement within the experimental group. Out of these, 7 (54 %) showed improvements in relation to an increase in PA before and after the intervention, 1 (80 %) only in relation to physical fitness, and 1 (8 %) only in relation to the quality of life (QoL) of its participants. In 4 studies (30 %), no differences were found between the mHealth intervention group and the face-to-face or mixed groups.
With reference to pathologies, the four studies related to heart disease presented differing results: in two of them, an improvement in PA was observed; in a third one, an improvement only in physical fitness (VO2max), and in the fourth one no differences between the study groups were reported. Nor were differences shown in terms of effectiveness of the mHealth intervention in the studies conducted with Parkinson’s patients, breast cancer survivors, or elderly women.
Adherence to physical activity
Eleven of the 13 studies (85 %) concluded that mHealth prescription is more effective within the control group, and 2 (15 %) that it shows no differences or it is worse in relation to face-to-face or mixed options. The results of one of these two studies could be affected by the unwillingness of the participants to use the technologies and communication channels proposed.
Workload variables used in the physical activity and physical exercise mHealth interventions
Regarding the variables used to assess workload, the results obtained showed that in the case of EL all the trials presented were based on quantifying the volume of steps or time (in minutes or hours) per day or per week, regardless of the disease and the population group in the sample. With respect to these quantitative variables, some cases offered variables related to intensity according to average values (5 out of 13, 38 %), considering this a defining variable of intensity. IL was taken as referring to work intensity in 5 out of 13 cases (38 %), of which 1 (7 % of the total) presented an objective physical variable, heart rate (HR), and 3 (23 % of the total) used an arbitrary unit (a.u.). Only 1 study (7 %) used a subjective variable: rate of perceived exertion (RPE).
Communication channels and technological resources
During the interventions, various mechanisms were established to communicate with the patients. Nine of the studies (69 %) were based on the use of mobile phones, 4 of these (31 %) using apps designed specifically for the intervention and 2 (15 %) by means of websites as an additional channel, while 6 (46 %) of the studies provided their patients with information by text messaging (SMS). Two studies (15 %) used Facebook for this purpose.
With regard to technological resources and tools for obtaining information, 8 of the 13 studies (62 %) were based on personalized questionnaires which made it possible to ascertain the patients’ initial and final state. Monitoring of the workloads used in the interventions was performed, in turn, in 8 of the studies (62 %): 3 using accelerometers (23 %), a further 3 with the FitBit® wristband and 2 with pedometers (15 %).
Discussion
Main findings
In this systematic review, we identified a greater effectiveness of and adherence to PA or PE prescription programs using digital devices than in those prescribed in person or without technological support. Workload was evaluated using variables of a quantitative and non-specific nature. Out of the variables used, EL was evaluated, in most cases, through total steps per day or minutes of PA or PE per day, while IL was evaluated in 5 of the 13 results obtained. The most commonly used technological resources were specific applications and the FitBit® wristband. The main communication channels were SMS and Facebook.
Effectiveness of PA and PE prescription for health (Muellmann et al., 2018) was assessed in terms of adherence to practicing PA and education in habits related to it (Wong et al., 2018), as well as the intent to improve and continue these habits (Shcherbina et al., 2019). Although the effectiveness criterion was not standardized, only 2 trials concluded that mHealth prescription was less effective or no better in the experimental groups than in the control groups (Quiñonez et al., 2016; Vorrink et al., 2016) and the remaining 11 concluded that mHealth prescription offered greater feasibility or effectiveness. These were notable for using and recording variables to determine whether or not there was an improvement in the patients’ satisfaction (Lee et al., 2018) and confidence (Partridge et al., 2017) in terms of QoL, as reflected in four of the studies presented here (Ellis et al., 2019; Mendoza et al., 2017; Uhm et al., 2017; Vorrink et al., 2016).
Workload can be evaluated using a range of variables, but the results obtained here show that in the case of EL all the studies presented are based on quantifying the volume of steps or time (in minutes or hours) per day or per week, regardless of the disease and the population group in the sample. With respect to these quantitative variables, some cases offered variables related to intensity according to average values (Duscha et al., 2018a; Duscha et al., 2018b; Ellis et al., 2019; Klausen et al., 2016; Mendoza et al., 2017), considering this a defining variable of intensity. For this purpose, IL should be applied (Soligard et al., 2016), and five studies do take it as a benchmark. One of them presented an objective physiological variable, HR (Klausen et al., 2016), identifying possible contraindications for adolescent patients with heart disease included in the study, and three used an arbitrary unit (a.u.), such as metabolic equivalents (METs) (Uhm et al., 2017; Vasankari et al., 2019; Vorrink et al., 2016). Only one study, with pregnant women, used a subjective variable: RPE (Choi et al., 2016). This individual variable was reliable in the health field (Utter et al., 2004) and was also applicable to a range of diseases (Fernández Lao et al., 2009).
In this context, the diagnosis determined the prescription (Paglialonga et al., 2018), but the individualization of the program (Duscha et al., 2018a) was defined both by patients’ habits with respect to PA and PE (Chodzko-Zajko et al., 2009) and by their initial physical capacity (Foster et al., 2017). For the purposes of the former, the studies presented here asked their participants to complete a series of validated questionnaires before starting the intervention, most notably the International Physical Activity Questionnaire (IPAQ) (Yu et al., 2015). However, none of them conducted a follow-up during the process in relation to PA or PE and issues of a behavioral or cognitive nature (Griffin et al., 2020), information that could be provided by the exercise questionnaire based on Prochaska and DiClemente’s transtheoretical model (Leyton et al., 2019) or a perceived quality of life questionnaire, such as COOP/WONCA (Van Weel et al., 2012). This factor was explored by four of the studies (Ellis et al., 2019; Mendoza et al., 2017a; Uhm et al., 2017; Vorrink et al., 2016), but only at the end of the intervention. The same pattern was repeated in relation to patients’ capacity. Only one study with heart patients (Vasankari et al., 2019) adapted PA prescription to each patient’s initial capacity. Ecological tests for this purpose could enable us to determine initial values in terms of volume, such as the Six-Minute Walk Test (Segura-Ortí & Martínez Olmos, 2009), which is reliable and adaptable to outpatients (Cabedo & Garcés, 2010), or the UKK test (Laukkanen et al., 2000). In any case, these must be able to be modulated to the prescribed needs, and the Talk Test (Reed & Pipe, 2014) may be an applicable tool in this area. Baseline improvement must be carried out during the process, as was done in a study with respiratory disease patients (Vorrink et al., 2016a) and another with Parkinson’s disease sufferers (Ellis et al., 2019).
The recording of these variables may be affected by the technological resource used during the intervention. In terms of quality and reliability, the outstanding option was the accelerometer (Rowlands & Eston, 2007), since it offered information on both the volume and the intensity of PA and PE. A limitation of this resource was that it requires a certain level of financial investment. It has been used in studies with respiratory pathologies (Vasankari et al., 2019; Vorrink et al., 2016) and with heart disease in adolescent patients (Klausen et al., 2012) as well as for the general population (Martin et al., 2015). A commercial product for recording PA and PE, the FitBit® wristband wearable tracking device, was used for recording load variables in studies with adolescent cancer survivors (Mendoza et al., 2017) and pregnant women (Choi et al., 2016), as well as older adults and people of advanced age with pulmonary diseases (Duscha et al., 2018b). This diversity of population groups is a notable feature (Powell & Deetjen, 2019; Shcherbina et al., 2019). The pedometer, a more affordable resource, was used in two cases: with adult Parkinson’s disease patients (Ellis et al., 2019) and with women who had survived breast cancer (Uhm et al., 2017).
However, the communication channel may circumscribe the capacity and individualization of the intervention if it does not allow records to be updated (Sohaib Aslam et al., 2020), which could improve patient involvement (Klausen et al., 2012), as well as enhancing the conduct of the intervention (Eckerstorfer et al., 2018) in terms of managing workload prescription. Most of the studies (9) developed smartphone apps to communicate and to update the work plan individually on the basis of the achievements attained or the activity carried out, and 8 used SMS text messages to update or recall the information. Two studies, one in young adults with no definite pathology (Hart et al., 2020) and another one in older adult cancer survivors (Mendoza et al., 2017), used the social media platform Facebook and two used a website specially created for the intervention (Quiñonez et al., 2016; Vorrink et al., 2016). One of those that used Facebook (Hart et al., 2020) also used quizzes to encourage adherence among its young adult users (Wong et al., 2018), but in no case did the studies make use of a current resource such as pervasive games (Santos et al., 2021). In this context, the greatest limitations of the studies were found, since the technical problems arising from connectivity, use and understanding of the technology have been described as one of the causes of patients abandoning interventions (Klausen et al., 2016; Vorrink et al., 2016). Practically all the trials used technological options to explain their programs, some to improve motivation though personalized messages with this aim (Shcherbina et al., 2019; Vasankari et al., 2019), but only two (Hart et al., 2020; Mendoza et al., 2017a) through socialization (Short et al., 2018), although in one of these cases, with older adult patients, the social network used —Facebook— was not in keeping with their generation. In this connection, only one of the studies, in which no differences were observed between the mHealth intervention and the control groups, presented the results as having been affected by the reluctance of the participants to use technological options and their low participation (Klausen et al., 2016). Similarly, another study, which obtained positive results in relation to an improvement in the values for the patients’ adherence to PA, highlights the need to modify communication channels, in view of how participation in the program decreased in the course of the intervention (Choi et al., 2016).
This systematic review has certain limitations inherent in the novelty of the subject addressed, as the number of clinical studies that met the inclusion criteria was limited. In addition, given the diversity of the pathologies and the ages and sociocultural circumstances of the population groups, the results presented are only exploratory. The low quality of the studies according to the SIGN criteria, few numbers of variables recorded, especially for IL, their heterogeneity, and the lack of consensus on the definition of concepts such as the effectiveness of the intervention mean that these data must be considered preliminary, while reflecting the need for future lines of research in this area.
In conclusion, mHealth interventions showed greater effectiveness and adherence compared to those prescribed in person or without technological support. However, workload was assessed by quantitative and non-specific parameters, and no interventions designed specifically for the needs or technological abilities of their target population were observed.
Practical Applications
A line of research has been established for improving PE prescription in the field of mHealth. Prescription of PA and PE must be validated with attention to individual needs, defined by pathologies, prescribed according to medical criteria and baseline physical fitness, and designed taking into account the patients’ technological abilities. Physical activity and physical exercise must be prescribed by professionals with a certified expertise in health and PA and PE adaptations. More effective PE prescription would make it possible to reach a larger population, as well as reduce treatment costs and manage the process more flexibly (Watson, 2020).
Funding
This study was funded by the Ministry of Science and Innovation (Ministerio de Ciencia e Innovación) of the Spanish Government (grant number: PID2019-107473RB-C21).The funders had no role in the study design, data collection and analysis, decision to publish, or preparation of the manuscript.
References
[1] Bull, F. C., Al-Ansari, S. S., Biddle, S., Borodulin, K., Buman, M. P., Cardon, G., Carty, C., Chaput, J.P., Chastin, S., Chou, R., Dempsey, P.C., Di Pietro, L. Ekelund, U., Firth, J., Friedenreich, C.M., Garcia, L., Gichu, M., Jago, R., Katzmarzy P.T., Lambert, E., Leitzmann, M., Milton, K., Ortega, F.B., Ranasinghe, Ch., Stamatakis, E., Tiedemann, A., Troiano, R.P., P van der Ploeg, H., Wari, V. & Willumsen, J. F. (2020). World Health Organization 2020 guidelines on physical activity and sedentary behaviour. British journal of sports medicine, 54(24), 1451-1462. http://dx.doi.org/10.1136/bjsports-2020-102955
[2] Cabedo, V. R., & Garcés, C. R. (2010). ¿Es útil la prueba de la marcha durante 6 min en Atención Primaria? Semergen, 36(5), 259–265. https://doi.org/10.1016/j.semerg.2009.06.002
[3] Capdevila, L., Rodas, G., Ocaña, M., Parrado, E., Pintanel, M., & Valero, M. (2008). Variabilitat de la freqüència cardíaca com a indicador de salut en esport: validació amb un qüestionari de qualitat de vida (SF-12). Apunts. Medicina de l’Esport, 158, 62–69. https://doi.org/10.1016/S1886-6581(08)70073-2
[4] Chodzko-Zajko, W. J., Proctor, D. N., Fiatarone Singh, M. A., Minson, C. T., Nigg, C. R., Salem, G. J., & Skinner, J. S. (2009). Exercise and physical activity for older adults. Medicine and Science in Sports and Exercise, 41(7), 1510–1530. https://doi.org/10.1249/MSS.0b013e3181a0c95c
[5] Choi, J., Lee, J. H., Vittinghoff, E., & Fukuoka, Y. (2016). mHealth Physical Activity Intervention: A Randomized Pilot Study in Physically Inactive Pregnant Women. Maternal and Child Health Journal, 20(5), 1091–1101. https://doi.org/10.1007/s10995-015-1895-7
[6] Duscha, B. D., Piner, L. W., Patel, M. P., Craig, K. P., Brady, M., McGarrah, R. W., Chen, C., & Kraus, W. E. (2018a). Effects of a 12-week mHealth program on peak VO 2 and physical activity patterns after completing cardiac rehabilitation: A randomized controlled trial. American Heart Journal, 199, 105–114. https://doi.org/10.1016/j.ahj.2018.02.001
[7] Duscha, B. D., Piner, L. W., Patel, M. P., Crawford, L. E., Jones, W. S., Patel, M. R., & Kraus, W. E. (2018b). Effects of a 12-Week mHealth Program on Functional Capacity and Physical Activity in Patients With Peripheral Artery Disease. American Journal of Cardiology, 122(5), 879–884. https://doi.org/10.1016/j.amjcard.2018.05.018
[8] Eckerstorfer, L. V., Tanzer, N. K., Vogrincic-Haselbacher, C., Kedia, G., Brohmer, H., Dinslaken, I., & Corcoran, K. (2018). Key elements of mHealth interventions to successfully increase physical activity: Meta-regression. JMIR MHealth and UHealth, 6(11). https://doi.org/10.2196/10076
[9] Ellis, T. D., Cavanaugh, J. T., DeAngelis, T., Kathryn Hendron, K., Thomas, C. A., Saint-Hilaire, M., Pencina, K., & Latham, N. K. (2019). Comparative effectiveness of mHealth-supported exercise compared with exercise alone for people with Parkinson disease: Randomized controlled pilot study. Physical Therapy, 2019; 99:203-216. https://doi.org/10.1093/ptj/pzaa061
[10] Fernández Lao, C., Valenza, M. C., García Ríos, M. C., & Valenza, G. (2009). Estudio de la disnea según la escala de Borg en un grupo de pacientes diagnosticados de asma bronquial que han seguido y recibido entrenamiento de fisioterapia respiratoria. Fisioterapia, 31(1), 12–16. https://doi.org/10.1016/j.ft.2008.01.004
[11] Foster, C., Rodriguez-Marroyo, J. A., & De Koning, J. J. (2017). Monitoring training loads: the past, the present, and the future. International journal of sports physiology and performance, 12(s2), S2-2. https://doi.org/10.1123/ijspp.2016-0388.
[12] Griffin, J. B., Struempler, B., Funderburk, K., Parmer, S. M., Tran, C., & Wadsworth, D. D. (2020). My Quest, a Community-Based mHealth Intervention to Increase Physical Activity and Promote Weight Loss in Predominantly Rural-Dwelling, Low-Income, Alabama Women. Family and Community Health, 43(2), 131–140. https://doi.org/10.1097/FCH.0000000000000251
[13] Harbour, R., & Miller, J. (2001). A new system for grading recommendations in evidence based guidelines. Bmj, 323(7308), 334-336. https://doi.org/10.1136/bmj.323.7308.334
[14] Hart, P. D., Benavidez, G., Potter, A., Rech, K., Budak, C. M., Auzenne, C., Failing, J., Kirkaldie, T., Lonebear, M., Miller, L., Auzenne, C., Failing, J., Kirkaldie, T., Lonebear, M., Miller, L., & Randomized, A. P. (2017). A Pilot Randomized Controlled Trial to Promote Physical Activity and Change Fitness Scores in Rural College Students: The Northern eHealth / mHealth Trial ( N-EMT ). 5(1), 43–48. https://doi.org/10.12691/jpm-5-1-6
[15] Hopewell, S., Clarke, M., Moher, D., Wager, E., Middleton, P., Altman, D.G., Schulz, K.F. & the CONSORT Group. (2008) CONSORT for Reporting Randomized Controlled Trials in Journal and Conference Abstracts: Explanation and Elaboration. PLOS Medicine 5(1): e20. https://doi.org/10.1371/journal.pmed.0050020
[16] Klausen, S. H., Andersen, L. L., Søndergaard, L., Jakobsen, J. C., Zoffmann, V., Dideriksen, K., Kruse, A., Mikkelsen, U. R., & Wetterslev, J. (2016). Effects of eHealth physical activity encouragement in adolescents with complex congenital heart disease: The PReVaiL randomized clinical trial. International Journal of Cardiology, 221, 1100–1106. https://doi.org/10.1016/j.ijcard.2016.07.092
[17] Klausen, S. H., Mikkelsen, U. R., Hirth, A., Wetterslev, J., Kjærgaard, H., Søndergaard, L., & Andersen, L. L. (2012). Design and rationale for the PREVAIL study: Effect of e-Health individually tailored encouragements to physical exercise on aerobic fitness among adolescents with congenital heart disease - a randomized clinical trial. American Heart Journal, 163(4), 549–556. doi.org/10.1016/j.ahj.2012.01.021
[18] Laukkanen, R. M. T., Kukkonen-Harjula, T. K., Oja, P., Pasanen, M. E., & Vuori, I. M. (2000). Prediction of change in maximal aerobic power by the 2-km walk test after walking training in middle-aged adults. International journal of sports medicine, 21(02), 113-116. https://doi.org/10.1055/s-2000-8872
[19] Lee, H., Uhm, K. E., Cheong, I. Y., Yoo, J. S., Chung, S. H., Park, Y. H., Lee, J. Y., & Hwang, J. H. (2018). Patient Satisfaction with Mobile Health (mHealth) Application for Exercise Intervention in Breast Cancer Survivors. Journal of Medical Systems, 42(12), 254. https://doi.org/10.1007/s10916-018-1096-1
[20] Leyton, M., Batista, M., Lobato, S., & Jimenez, R. (2019). Validación del cuestionario del modelo transteórico del cambio de ejercicio físico. Revista internacional de medicina y ciencias de la actividad fisica y del deporte, 19(74), 329-350. http://doi.org/10.15366/rimcafd2019.74.010
[21] Martín-Guillaumes, J., Caparrós, T., Cruz-Puntí, D., Montull, L., Orriols, G., & Capdevila, L. (2018). Psychophysiological monitoring of the recovery process in the elite athletes of the Spanish National Ski Mountaineering Team through the RMSSD and the subjective perception of recovery. Revista Iberoamericana de Psicologia del Ejercicio y el Deporte, 13(2), 219-223.
[22] Martin, S. S., Feldman, D. I., Blumenthal, R. S., Jones, S. R., Post, W. S., McKibben, R. A., Michos, E. D., Ndumele, C. E., Ratchford, E. V., Coresh, J., & Blaha, M. J. (2015). mActive: A randomized clinical trial of an automated mHealth intervention for physical activity promotion. Journal of the American Heart Association, 4(11). https://doi.org/10.1161/JAHA.115.002239
[23] Mendoza, J. A., Baker, K. S., Moreno, M. A., Whitlock, K., Abbey-Lambertz, M., Waite, A., Colburn, T., & Chow, E. J. (2017). A Fitbit and Facebook mHealth intervention for promoting physical activity among adolescent and young adult childhood cancer survivors: A pilot study. Pediatric Blood and Cancer, 64(12), 1–9. https://doi.org/10.1002/pbc.26660
[24] Moreno, J., Cervantes, J., Parrado, E., & Ll, C. (2013). Variabilidad de la frecuencia cardíaca y perfiles psicofisiológicos en deportes de equipo de alto rendimiento. Revista de Psicologia del Deporte, 22(2), 345-352. https://ddd.uab.cat/record/114200
[25] Muellmann, S., Forberger, S., Möllers, T., Bröring, E., Zeeb, H., & Pischke, C. R. (2018). Effectiveness of eHealth interventions for the promotion of physical activity in older adults: A systematic review. Preventive Medicine, 108(November 2017), 93–110. https://doi.org/10.1016/j.ypmed.2017.12.026
[26] Mujika, I. (2017). Quantification of training and competition loads in endurance sports: methods and applications. International journal of sports physiology and performance, 12(s2), S2-9. https://doi.org/10.1123/ijspp.2016-0403
[27] Muyor, J. M. (2013). Exercise intensity and validity of the ratings of perceived exertion (Borg and OMNI Scales) in an indoor cycling session. Journal of Human Kinetics, 39(1), 93–101. https://doi.org/10.2478/hukin-2013-0072
[28] Page, M. J., McKenzie, J. E., Bossuyt, P. M., Boutron, I., Hoffmann, T. C., Mulrow, C. D., Shamseer, L., Tetzlaff, J.M., Akl, E.A., Brennan, S.E., Chou, R., Glanville, J., Grimshaw, J.M., Hróbjartsson, A., Lalu, M.M., Li, T., Loder, E.W., Mayo-Wilson, E., McDonald, S., McGuinness; L.A., Stewart, L.A., Thomas, J., Tricco, A.C., Welch, V.A., Whiting, P. & Moher, D. (2021). The PRISMA 2020 statement: an updated guideline for reporting systematic reviews. Systematic reviews, 10(1), 1-11. https://doi.org/10.1186/s13643-021-01626-4
[29] Paglialonga, A., Lugo, A., & Santoro, E. (2018). An overview on the emerging area of identification, characterization, and assessment of health apps. Journal of Biomedical Informatics, 83(May), 97–102. https://doi.org/10.1016/j.jbi.2018.05.017
[30] Partridge, S. R., McGeechan, K., Bauman, A., Phongsavan, P., & Allman-Farinelli, M. (2017). Improved confidence in performing nutrition and physical activity behaviours mediates behavioural change in young adults: Mediation results of a randomised controlled mHealth intervention. Appetite, 108, 425–433. https://doi.org/10.1016/j.appet.2016.11.005
[31] Powell, J., & Deetjen, U. (2019). Characterizing the digital health citizen: Mixed-methods study deriving a new typology. Journal of Medical Internet Research, 21(3). https://doi.org/10.2196/11279
[32] Quiñonez, S. G., Walthouwer, M. J. L., Schulz, D. N., & De Vries, H. (2016). MHealth or eHealth? Efficacy, use, and appreciation of a web-based computer-tailored physical activity intervention for Dutch adults: A randomized controlled trial. Journal of Medical Internet Research, 18(11), 1–12. https://doi.org/10.2196/jmir.6171
[33] Reed, J. L., & Pipe, A. L. (2014). The talk test: A useful tool for prescribing and monitoring exercise intensity. Current Opinion in Cardiology, 29(5), 475–480. https://doi.org/10.1097/HCO.0000000000000097
[34] Rethlefsen, M. L., Kirtley, S., Waffenschmidt, S., Ayala, A. P., Moher, D., Page, M. J., & Koffel, J. B. (2021). PRISMA-S: an extension to the PRISMA statement for reporting literature searches in systematic reviews. Systematic reviews, 10(1), 1-19. https://doi.org/10.1186/s13643-020-01542-z
[35] Rowlands, A. V., & Eston, R. G. (2007). The measurement and interpretation of children’s physical activity. Journal of Sports Science and Medicine, 6(3), 270–276.
[36] Santos, L. H. D. O., Okamoto, K., Otsuki, R., Hiragi, S., Yamamoto, G., Sugiyama, O., Aoyama, T., & Kuroda, T. (2021). Promoting Physical Activity in Japanese Older Adults Using a Social Pervasive Game: Randomized Controlled Trial. JMIR Serious Games, 9(1), e16458. https://doi.org/10.2196/16458
[37] Segura-Ortí, E., & Martínez Olmos, F. J. (2009). Análisis de correlaciones entre los resultados de una prueba de esfuerzo y de la prueba de 6 minutos marcha en población sana. Fisioterapia, 31(6), 241–247. https://doi.org/10.1016/j.ft.2009.05.003
[38] Shcherbina, A., Hershman, S. G., Lazzeroni, L., King, A. C., O’Sullivan, J. W., Hekler, E., Moayedi, Y., Pavlovic, A., Waggott, D., Sharma, A., Yeung, A., Christle, J. W., Wheeler, M. T., McConnell, M. V., Harrington, R. A., & Ashley, E. A. (2019). The effect of digital physical activity interventions on daily step count: a randomised controlled crossover substudy of the MyHeart Counts Cardiovascular Health Study. The Lancet Digital Health, 1(7), e344–e352. https://doi.org/10.1016/S2589-7500(19)30129-3
[39] Short, C. E., Finlay, A., Sanders, I., & Maher, C. (2018). Development and pilot evaluation of a clinic-based mHealth app referral service to support adult cancer survivors increase their participation in physical activity using publicly available mobile apps. BMC Health Services Research, 18(1), 1–11. https://doi.org/10.1186/s12913-017-2818-7
[40] Silva, B. M. C., Rodrigues, J. J. P. C., de la Torre Díez, I., López-Coronado, M., & Saleem, K. (2015). Mobile-health: A review of current state in 2015. Journal of Biomedical Informatics, 56, 265–272. https://doi.org/10.1016/j.jbi.2015.06.003
[41] Sohaib Aslam, A., van Luenen, S., Aslam, S., van Bodegom, D., & Chavannes, N. H. (2020). A systematic review on the use of mHealth to increase physical activity in older people. Clinical EHealth, 3, 31–39. https://doi.org/10.1016/j.ceh.2020.04.002
[42] Soligard, T., Schwellnus, M., Alonso, J.-M., Bahr, R., Clarsen, B., Dijkstra, H. P., Gabbett, T., Gleeson, M., Hägglund, M., Hutchinson, M. R., Janse van Rensburg, C., Khan, K. M., Meeusen, R., Orchard, J. W., Pluim, B. M., Raftery, M., Budgett, R., & Engebretsen, L. (2016). How much is too much? (Part 1) International Olympic Committee consensus statement on load in sport and risk of injury. British Journal of Sports Medicine, 50(17), 1030–1041. https://doi.org/10.1136/bjsports-2016-096581
[43] Uhm, K. E., Yoo, J. S., Chung, S. H., Lee, J. D., Lee, I., Kim, J. Il, Lee, S. K., Nam, S. J., Park, Y. H., Lee, J. Y., & Hwang, J. H. (2017). Effects of exercise intervention in breast cancer patients: is mobile health (mHealth) with pedometer more effective than conventional program using brochure? Breast Cancer Research and Treatment, 161(3), 443–452. https://doi.org/10.1007/s10549-016-4065-8
[44] Utter, A. C., Robertson, R. J., Green, J. M., Suminski, R. R., McAnulty, S. R., & Nieman, D. C. (2004). Validation of the adult OMNI Scale of Perceived Exertion for walking/running exercise. Medicine and Science in Sports and Exercise, 36(10), 1776–1780. https://doi.org/10.1249/01.MSS.0000142310.97274.94
[45] Vasankari, V., Halonen, J., Husu, P., Vähä-Ypyä, H., Tokola, K., Suni, J., Sievänen, H., Anttila, V., Airaksinen, J., Vasankari, T., & Hartikainen, J. (2019). Personalised eHealth intervention to increase physical activity and reduce sedentary behaviour in rehabilitation after cardiac operations: Study protocol for the PACO randomised controlled trial (NCT03470246). BMJ Open Sport and Exercise Medicine, 5(1), 1–8. https://doi.org/10.1136/bmjsem-2019-000539
[46] Vorrink, S. N. W., Kort, H. S. M., Troosters, T., Zanen, P., & Lammers, J.-W. J. (2016). Efficacy of an mHealth intervention to stimulate physical activity in COPD patients after pulmonary rehabilitation. The European Respiratory Journal, 48(4), 1019–1029. https://doi.org/10.1183/13993003.00083-2016
[47] Watson, M. (2020). Special Issue on eHealth Innovations and Psycho-oncology. Psycho-Oncology, 29(1), 3. https://doi.org/10.1002/pon.5308
[48] Van Weel, C., König-Zahn, C., Touw-Otten, F. W. M. M., van Duijn, N. P., & Meyboom-de Jong, B. (2012). Measuring functional status with the COOP/WONCA Charts. A manual. Groningen, the Netherlands: Noordelijk Centrum voor GEzondheidsvraagstukken (NCG)/Northern Centre of Health Care Research (NCH). Series, (7)
[49] Wong, E. M., Chair, S. Y., Leung, D. Y., Sit, J. W., & Leung, K. P. (2018). Home-based interactive e-health educational intervention for middle-aged adults to improve total exercise, adherence rate, exercise efficacy, and outcome: a randomised controlled trial. Hong Kong Medical Journal = Xianggang Yi Xue Za Zhi, 24 Suppl 2(1), 34–38. http://www.ncbi.nlm.nih.gov/pubmed/29938656
[50] WHO Global Observatory for eHealth. (2011). mHealth: new horizons for health through mobile technologies: second global survey on eHealth. World Health Organization. https://apps.who.int/iris/handle/10665/44607
[51] Yu, C. an, Rouse, P. C., Veldhuijzen Van Zanten, J. J. C. S., Ntoumanis, N., Kitas, G. D., Duda, J. L., & Metsios, G. S. (2015). Subjective and objective levels of physical activity and their association with cardiorespiratory fitness in rheumatoid arthritis patients. Arthritis Research and Therapy, 17(1), 1–7. https://doi.org/10.1186/s13075-015-0584-7
[52] Zenko, Z., & Ekkekakis, P. (2015). Knowledge of Exercise Prescription Guidelines Among Certified Exercise Professionals. Journal of Strength and Conditioning Research, 29(5), 1422–1432. https://doi.org/10.1519/JSC.0000000000000771
ISSN: 2014-0983
Received: June 6, 2022
Accepted: September 19, 2022
Published: January 1, 2023
Editor: © Generalitat de Catalunya Departament de la Presidència Institut Nacional d’Educació Física de Catalunya (INEFC)
© Copyright Generalitat de Catalunya (INEFC). This article is available from url https://www.revista-apunts.com/. This work is licensed under a Creative Commons Attribution-NonCommercial-NoDerivatives 4.0 International License. The images or other third party material in this article are included in the article’s Creative Commons license, unless indicated otherwise in the credit line; if the material is not included under the Creative Commons license, users will need to obtain permission from the license holder to reproduce the material. To view a copy of this license, visit https://creativecommons.org/licenses/by-nc-nd/4.0/deed.en