Validation of an instrument to qualify football competence via WyScout
*Corresponding author: R. Sánchez-López rsanchez051@ikasle.ehu.eus
Cite this article
Sánchez-López, R., Echeazarra, I. & Castellano, J. (2023). Validation of an instrument to qualify Football Competence in professional players via WyScout data provider. Apunts Educación Física y Deportes, 154, 83-94. https://doi.org/10.5672/apunts.2014-0983.es.(2023/4).154.08
Abstract
The aim of this work was to design and validate an instrument (TOPSTATS) to rate, classify and compare the performance of professional players based on the Wyscout data provider. Content validity through consultation with three experts showed considerable agreement using Fleiss’ kappa index (k = .691). Extracting data on players’ play in the 2019-2020 season in La Liga and the English Premier League, criterion validity was calculated by relating the total player scores obtained in TOPSTATS to those in SofaScore. Pearson’s correlation showed a significant association in all playing positions (r = 0.3-0.88, p < .05). The same procedure was used to ensure construct validity, relating the players’ total scores to their market value. In this case, Pearson’s correlation showed a significant association in 17 of the 24 playing positions (r = 0.36-0.80; p < .05). It was concluded that TOPSTATS showed optimal validity values. It is an instrument capable of comparing the football competence shown by players in their play during the same competition, according to their playing position. To this end, the tool allows for the agile and semi-automatic calculation of an overall performance index obtained from the interaction and weighting of variables containing data provided by Wyscout, which, being a subscription platform, has coverage in more than 200 competitions.
Introduction
Sports data analysis has long been of interest (Anderson & Sally, 2014; Bornn et al., 2018), but it is only in recent years that football statistics have been developed, thanks to technologies that provide large streams of highly reliable data extracted on a match-by-match basis (Pappalardo et al., 2019b). Unfortunately, the use and manipulation of this data is not an easy endeavour if the aim is to assess the football competence (Parlebas, 2018) exhibited by players during their play, as there is no consolidated and widely accepted metric to measure the quality of performance in all its facets (Pappalardo et al., 2019a). This is because football is a highly complex sport, which requires a multidimensional approach, as players’ motor responses are dynamic and non-linear (Garganta & Gréhaigne, 1999; Gréhaigne et al., 1997; Ric et al., 2016).
Players’ motor responses are shaped by the behaviours they exhibit in accordance with their socio-motor role. The role of each player is a specific socio-motor status (Parlebas, 2001), which changes during the game. Each player develops his or her behaviours based on the particular approach he or she takes to his or her role (Lasierra, 1993). In other words, from the actions of the socio-motor subroles, which result in each of the possible behaviours that the player can develop during the game (Hernández Moreno, 1995), with each behaviour representing a particular modality of the generic concept of “motor action” (Parlebas, 2023). The efficacy of the behaviours displayed by each player during his or her play in a given event or set of events determines his or her performance. Whilst performance is concrete and temporary, competence is an overarching concept that deals with lasting and stable performance over time as a result of learning.
Providers essentially provide two types of data: “tracking” and “eventing”. Tracking data provides data on the exact position of the player on the field (Otero-Saborido et al., 2021), allowing for the assessment of emergent behaviours through the player’s position and movement tendencies that result from interaction with teammates and opponents. Based on these positional data, scientific studies have been carried out on football training (Coutinho et al., 2022; Errekagorri et al., 2020) and elite football (Castellano & Echeazarra, 2019). Eventing data consists of recording and labelling the actions carried out by the player, as well as their effectiveness, usually in relation to the ball (Otero-Saborido et al., 2021). Using the Wyscout provider, several recently published papers can be retrieved (Díez et al., 2021; Izzo et al., 2020; Zeng & Pan, 2021).
The performance of players with respect to a variable can be compared within the eventing platforms offered by data providers. It is also possible to use scatter plots to compare variables in pairs, or even to agglomerate several metrics into one graph, which is usually presented in the form of a radar. However, none of these options tend to answer questions such as: which player is performing best in the league, which player should be signed; and player selection is a key factor in the success of any competition (Partovi & Corredoira, 2002). In other words, if a striker scores a lot of goals, perhaps his/her overall performance could be considered high, because scoring is a very important variable, but it is not the only factor to consider. Similarly, if a winger is a highly effective dribbler, or a centre-back an interceptor, it cannot be assumed that their overall performance will be high, since performance in football, as has been noted, is a multifactorial construct. In this sense, it is evident that within a playing position there are some actions that are more important than others, either because they occur more frequently or because they are particularly relevant.
Currently, large amounts of data are collected, although in many cases the validity of the metrics, i.e. how they are linked to success or whether they allow different levels of performance to be defined, is unknown (Castellano & Clemente, 2020). It is clear that there is a progressive shift from the classic metrics offered by suppliers to more advanced and contextualised metrics. In this way, game play, traditionally identified from the player’s relationship with the ball, is progressively being understood as the result of the functional interaction between the player and his or her environment with a certain purpose (Araújo, 2005). Through sufficiently large databases, in which play according to different variables has been recorded, analysts can obtain overall rankings and ratings of players through the evaluation of all these actions (Berrar et al., 2019). Therefore, it is important to assess the validity of performance ratings and indices quantitatively and comprehensively, through datasets created with the help of experts (Pappalardo et al., 2019a).
In short, given the need for calculating a global performance indicator, a product of the volume and effectiveness of the most representative actions that players perform according to their playing position, the aim of this work was to design and validate an instrument that would allow the performance of professional players to be rated, classified and compared, understood as the footballing competence they show in their play, based on the Wyscout data provider.
Method
Design
The present work responded to an instrumental study (León & Montero, 2007), aimed at the design and validation of a tool for the qualification, classification and comparison of football competence in professional football players. It should be noted that this study did not address the process of obtaining evidence of reliability on the data extracted from the Wyscout platform, as the results found in a previous study (Pappalardo et al., 2019a), which replicated the protocol used to ensure the reliability of Opta data (Liu et al., 2013), were used to confirm the reliability of the data.
Participants
For the design and validation of the TOPSTATS instrument, two football experts, together with a researcher, contributed their knowledge in four consultation and discussion sessions. The two football experts had more than 10 years of experience in analysing the Spanish Primera División and the English Premier League, respectively.
Instrument Design
The TOPSTATS instrument was designed using Excel 2013 software, factoring in the 111 variables provided by the supplier Wyscout at the time of validation of the tool. Ultimately, it consists of a set of Excel files designed ad hoc that separately represent those playing positions that, in the opinion of the experts involved in the validation process of the instrument, are most commonly encountered in the teams. There are nine of these: goalkeeper, central defender, full-back (right and left), defensive midfield, box-to-box, creative midfield, wide midfield (right and left), winger (right and left) and striker. For each position, the overall performance of the players who play in that position is analysed, based on the interaction of the 12 most representative variables, as judged by the experts.
Validity of Instrument
Any instrument that claims to be scientific, regardless of its type and objective, must undergo a process of validation. Traditionally, three types of validity are recognised: content, construct and criterion validity (Cronbach & Meehl, 1955). Content validity qualitatively assesses whether the instrument measures what it is intended to measure. In this case, in order to assess the football competence of a player, the most representative variables must be selected according to the position played. In order to do so, it appears necessary to rely on expert judgement. Another of the keys to validating this type of instrument is criterion validity, i.e. comparing the results obtained in the instrument to be validated with an external criterion that aims to measure the same thing (Hernández et al., 2010), in order to ensure that there are no significant differences between the two measurement criteria. Finally, construct validity can be expressed in different ways, using the perspective of discriminant validity in this kind of instrument, as it allows for validating whether the instrument is able to distinguish between individuals who are expected to be different (Carvajal et al., 2011). As for the reliability of the data, it is directly dependent on the suppliers, unless ad hoc tools designed for this purpose are used. This may be common in analytical departments that aim to collect data in a certain way from observation. If so, it is necessary to ensure that the tool is used homogeneously by all analysts involved in the process, so that it is always measured in the same way.
Procedure
The instrument design and validation process was carried out in six stages (see Figure 1), following the procedure established in other studies that have validated tools recently (Sánchez-López et al., 2021, 2023c, 2023b): (a) literature review and provisional design of the instrument identifying seven possible playing positions, with their respective action zones and associated metrics, (b) consultation and discussion with experts to approve or modify the most commonly played positions in the teams, (c) content validation of the instrument based on the agreement among experts of the 12 most representative variables in each position studied, (d) consultation and discussion with experts to adjust the weighting of the variables, taking the data extracted from La Liga and the Premier League as a reference, as well as the action zones to be filtered for each playing position, (e) criterion validation, and (f) construct validation of the instrument.
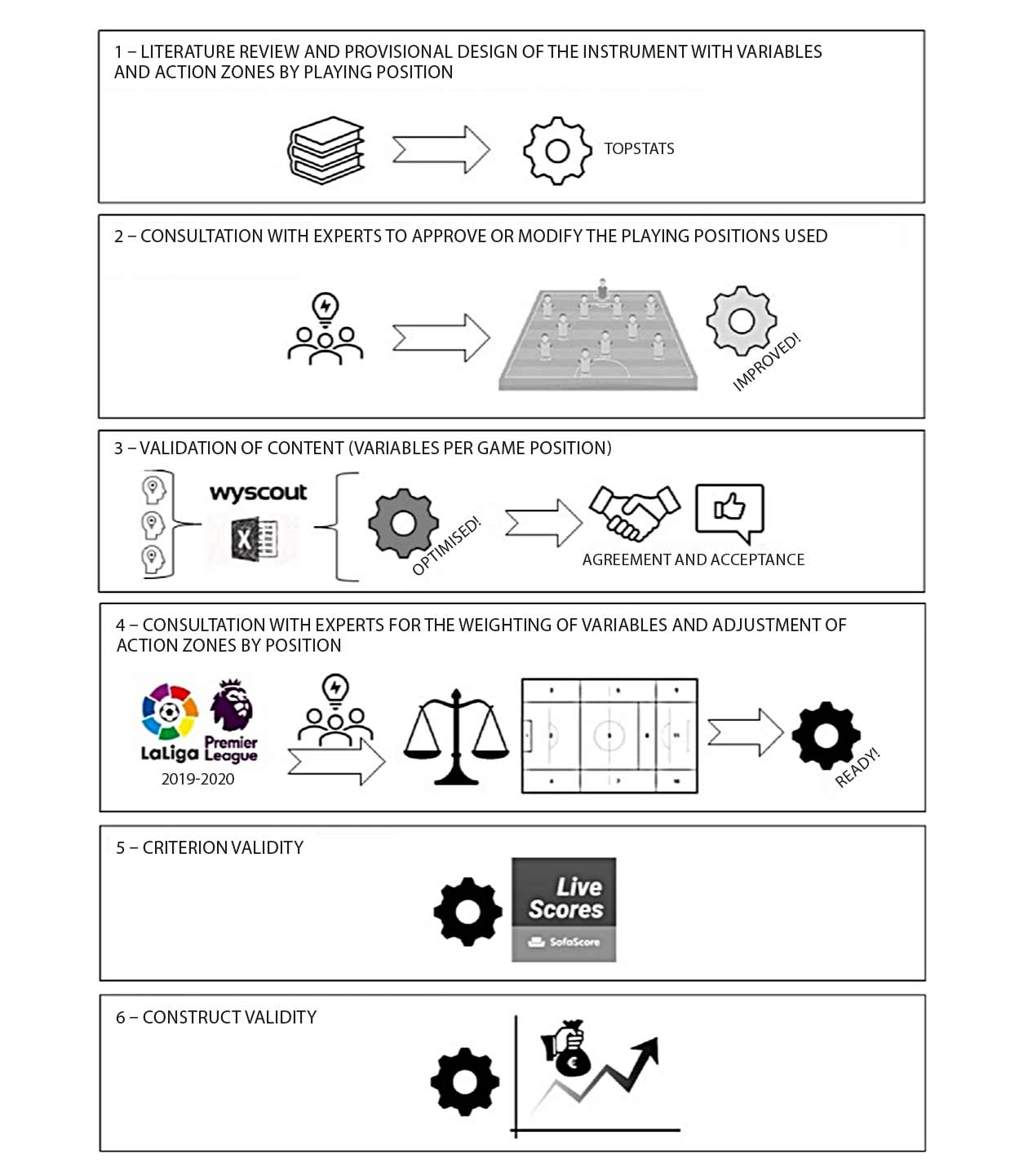
In the first stage, a literature review was carried out on studies that analysed the most significant technical-tactical variables in each playing position (Dellal et al., 2010, 2011; Firiteanu Vasile, 2013; Hughes et al., 2012; Van Lingen, 1997; Wiemeyer, 2003; Yi et al, 2018) and their spatio-temporal data (Konefał et al., 2019; Pappalardo et al., 2019b), as well as studies aimed at designing tools that assess sport performance from supplier data (Brooks et al., 2016; Duch et al., 2010; Pappalardo et al., 2019a).
Based on this review, the provisional tool was designed, initially with seven playing positions (goalkeeper, centre-back, right/left full-back, defensive midfield, attacking midfield, right/left wing, striker). Later, two new playing positions were to be included. For each playing position, the 12 most decisive variables in the opinion of the researcher were selected. This selection of variables was determined by the metrics offered by the subscription provider Wyscout. In this case, Wyscout was able to provide a total of 111 variables on the player at the time of validation of the tool. Data for which can be downloaded from the “advanced search” option, filtering by league, period, position, as well as by any variable that is intended to drive the search.
The provisional selection of variables for each playing position was not shared with the experts until the third stage, and these data were used for comparison with the data extracted from the experts’ criteria to validate the tool. In this phase, the action zones of each playing position were also provisionally determined according to the positional filter offered by Wyscout. This selection was to be fine-tuned in the fourth stage through discussion with experts.
Based on the aforementioned prior selection of variables for each position, files were designed in Excel for the analysis and processing of the data obtained. These would be adapted during the process until the final version was obtained, in accordance with each game position. The software focuses on the data of the 12 variables selected for each position, establishing 7 intervals according to the minimum and maximum data for each variable studied. Subsequently, those players who meet the positional filtering are ranked on the corresponding interval for each variable using a Likert scale 1-7, with 1 being not competent at all and 7 being very competent. In this way, the variables are standardised so that they all “speak the same language”. Finally, a report displaying the performance index of the entire sample of players analysed is obtained from the sum of the 12 ratings obtained, which are weighted according to their level of relevance in the playing position.
In the second stage, in a first discussion session, the researcher presented the provisional playing positions to the experts, resulting in a consensus on including new playing positions to better reflect the most common positions in a football team. Accordingly, nine playing positions were considered (goalkeeper, centre-back, right/left full-back, defensive midfield, box-to-box, creative midfield, right/left wing, right/left winger, striker). Once this was done, and to conclude the first discussion session, the researcher agreed to send a list of 108 variables to the experts so that they could independently select the most relevant variables by playing position, before scheduling a new discussion session.
In the third stage, the experts had to independently indicate the 12 variables they considered most relevant for each of the playing positions when assessing the football competence of the players playing in those positions. A selection of 102 of the 111 variables offered by Wyscout was allowed, as player name was discarded because it is the representative variable of the player, the specific position, as this variable was used for filtering, the market value that would be used later to carry out the construct validity process of the tool, as well as other variables that did not offer quantitative or relevant data in the opinion of the researcher (current team, contract expiration, country of birth, passport, foot, on loan). It was agreed that other variables which, although not directly related to game assessment, might be important in determining player performance, should not be ruled out. For example, in reference to the variable minutes played, those players with the highest level of participation in their teams tend to be the most competent players. The variable age could also influence medium to long-term performance in younger players. The height variable can be a determining factor in the position of goalkeeper. They were also provided with the Wyscout event glossary describing each of the variables (https://dataglossary.wyscout.com/).
Excel software was used to carry out this process. The researcher also had to perform this task with the new game positions validated during the first discussion session. Once this was done, and the researcher had received the files, content validity was calculated. In this way, the concordance of the two experts’ selections was used, as well as the provisional selection made by the researcher, comparing the data jointly through Fleiss’ kappa coefficient, as well as in pairs, using Cohen’s kappa coefficient. Once sufficiently high agreement was obtained, a second discussion was held with the two experts, and they were shown the researcher’s provisional selection of variables for each position, as well as the two selections made by the experts themselves. Once this was done, all variables that had been selected by the three experts for each position were approved, while those variables that had been selected by one or two experts were discussed until consensus was reached on their inclusion/exclusion, and the 12 most representative variables for each game position were determined. For the position of striker it was decided that a metric resulting from the number of goals divided by the number of shots would be included.
In the fourth stage, a third and fourth discussion session were held with the two experts to weight the 12 variables selected for each position. For this purpose, data from the 2019-2020 Spanish Primera División season (matchdays 1 to 27) and the English Premier League (matchdays 1 to 29) were used, and the discussion began with the variables unweighted, i.e. they all had the same weight when it came to assessing player performance. It was decided that this process should be carried out jointly, rather than independently, as both the experts and the researcher could contribute their ideas and knowledge on how each of the variables could influence one another, taking into account the direct dependence that they would always have on the desired player profile and the game style in which they are being assessed.
Table 1 shows the list of selected variables and their weights. In parallel to this process, and as mentioned in stage 1, the action zones of each playing position were adjusted through the analysis of the players that appeared or disappeared when any of the zones were selected or not in the filter offered by Wyscout. In this way, the most relevant zones for each playing position were determined. Figure 2 shows the filtered zones for each playing position after the above mentioned process.

Table 1
Variables selected for each position and their weighting based on expert consensus.
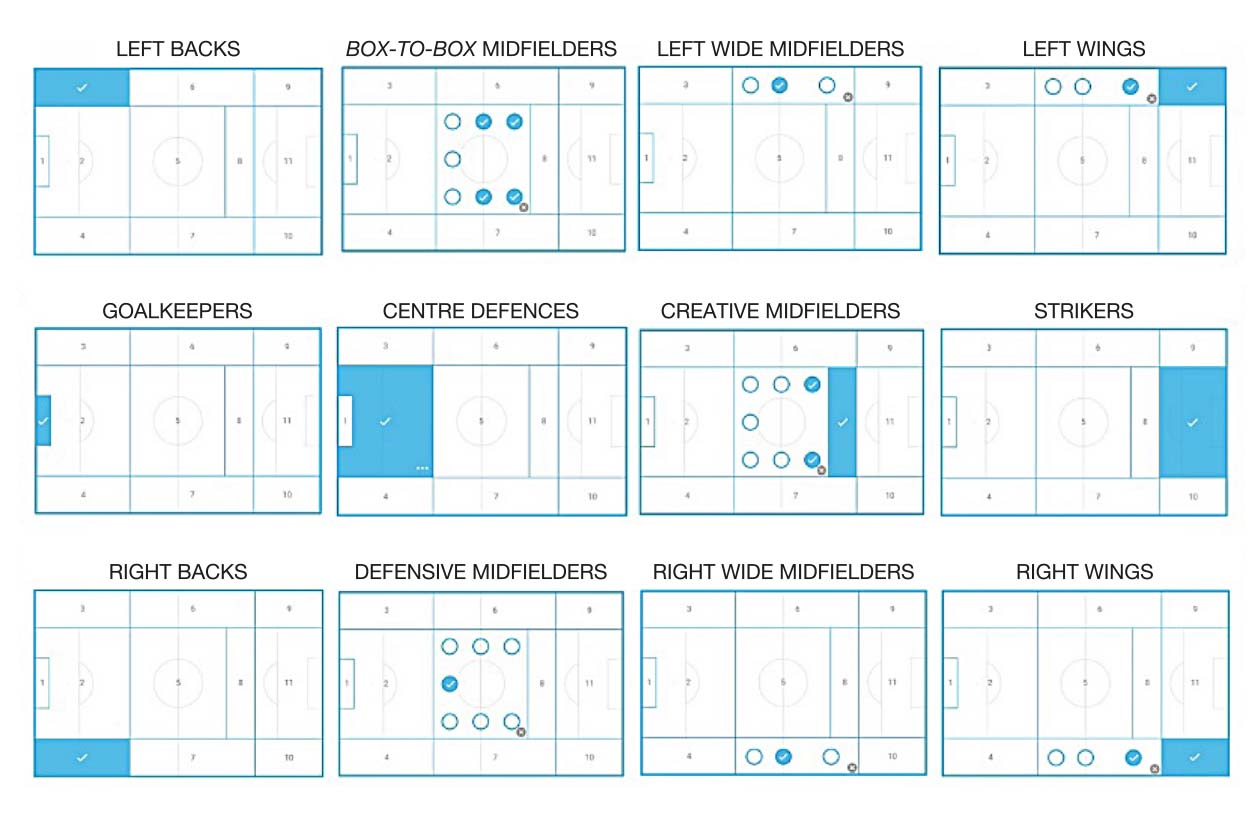
In the fifth stage, the criterion validity of the instrument was calculated. This estimates the degree of agreement with some external criterion that purports to measure the same thing. For this purpose, player performance indices obtained in TOPSTATS from the weighted total score in each playing position were compared with player scores in SofaScore. The player scores on SofaScore are calculated objectively using data from the provider Opta.
In the sixth stage, to estimate construct validity, the discriminant validity perspective was used (Carvajal et al., 2011), which measures the degree to which the instrument is able to distinguish between groups of individuals who are expected to be different (McDowell & Newell, 1996), due to their characteristics or performance (Thomas et al., 2011). In this particular case, the market value of the players was used to determine if this related to the overall TOPSTATS score.
Statistical Analysis
In order to calculate content validity, expert consultation was needed, as described in the third step of the procedure. Through this process, the Fleiss kappa index from the Excel “Real Statistics” add-in was used to calculate the degree of agreement between the 3 experts when selecting the 12 most relevant variables in each of the game positions. This procedure allows the degree of agreement between two or more observers to be obtained. Although it is usually used as a measure of reliability, given the uniqueness of this study, it was considered the best way to undertake this step for the content validity of the tool, as it was intended to verify whether each position was measuring what it was intended to measure, according to the variables selected by the experts, given that in most sports it is found that important performance indicators vary from one coach to another (Hughes et al., 2012). Similarly, as a supplementary procedure, SPSS v.19 software was used, using Cohen’s (1988) kappa agreement index by pairs of observers, allowing the same final result to be arrived at in a different way.
In the fifth and sixth stages of the procedure, the Pearson correlation was used to determine the criterion and construct validity of the tool by relating the total scores of the players obtained in TOPSTATS to the SofaScore and to their market value, respectively.
Results
For content validity, a Fleiss kappa index (k = .691) was obtained, indicating considerable agreement between the three observers according to the interpretation scale proposed by Landis & Koch (1977). For observer-pair agreement, a similar average (k = .691) was obtained from Cohen’s kappa index. The pairwise concordance can be seen in table 2.

For criterion validity, the total player scores obtained in TOPSTATS were related to those of Sofascore through Pearson’s correlation index (see table 3), with significant evidence of association (r = 0.3–0.88; p < .05) in all playing positions.

Table 3
Pearsoncorrelation between Overall Performance Index (total weighted) in TOPSTATS and SofaScore ratings of players with more than 800 minutes played in the 2019-20 League and Premiership.
Finally, to estimate the construct validity of the tool, the total player scores obtained in TOPSTATS were correlated with their market value extracted from Wyscout at the time of the analysis. In this case, there was significant evidence of association in 17 of the 24 playing positions (r = 36-80; p < .05), excluding the positions of left-back and box-to-box midfield in the Spanish La Liga, and right and left wings in both competitions (see table 4).

Table 4
Pearson Correlation between Overall Performance Index (total weighted) in TOPSTATS and market value of players with more than 800 minutes played in La Liga and Premier League 2019-20.
Discussion
The aim of the present study was to design and validate an instrument to rate, classify and compare the overall performance of professional players, understood as the football competence they show throughout their play, based on the Wyscout data provider. The developed instrument analyses data extracted from the Wyscout provider in a semi-automatic way, and provides a player rating, in the form of an overall performance index, according to their position and in relation to players playing in the same position and in the same league. In this way, comparisons between players can be made quickly, which is crucial when working in sports clubs where time is of the essence and analysis processes are carried out at a dizzying pace. In addition, this process can be carried out in more than 200 competitions that are included in Wyscout data coverage.
Football competence has been studied in recent works using an observational system (Sánchez-López et al., 2021), or by describing collective behaviours according to the level of football competence shown by the participants (Nieto et al., 2022). As a central concept of this work, in the face of very different teams, it is revealed by the player’s ability to adapt to the behaviour of the other players, to participate in a collective strategy, to show empathy and anticipation in the position, and to be successful in the behaviours developed during the match (Parlebas, 2018). This is why play and competition are two inseparable terms, as play is the product of competition, and performance during play is the best indicator of the players’ competence.
Ensuring the validity of any instrument that aims to assess the performance of players is a necessary step in order to guarantee the quality of the assessment. One of the problems that often arises when trying to obtain evidence of content validity through expert knowledge is that a list of the correct content of the phenomenon to be measured is often not available and therefore has to be established (Carvajal et al., 2011). In the case of this study, the principal researcher provided a detailed list of all the metrics that can be extracted from Wyscout, and the experts had to establish which metrics were the most relevant for each playing position. This part of the research was carried out with great care and patience, in order to ensure expert consensus on the selection of the variables that define each positional profile, as well as the weights associated with each variable. The experts’ knowledge of the performance of players in both La Liga and the Premier League allowed them to adjust these weights in order to obtain total scores in line with reality.
In order to obtain evidence of criterion validity, scores from the SofaScore platform were used as external criteria that purported to measure the same thing. These scores are the result of the data processing provided by the Opta provider, which was found to be reliable in a previous study (Liu et al., 2013). Sofascore, at the time of publication of this paper, continues to increase its data coverage, but falls short of Wyscout’s data coverage, so a tool such as TOPSTATS could be used to obtain global performance indices across a larger volume of countries and divisions. For example, in the case of Spain, performance indices could be obtained in divisions such as the 1st RFEF or 2nd Spanish RFEF which, at the date of submission of this paper, are not supported by Sofascore.
With respect to construct validity, it could be seen that in 7 of the 24 positions analysed, there was no relationship between the rating of the players and market value. This was because market value is not only determined by the performance of the players but also by their potential and future possibilities. Age is therefore a variable that can skew results in certain positions, as experienced players approaching the final years of their careers had very low market values. For example, Joaquín and Cazorla in La Liga, both performing at a very high level in midfield positions. It is also the case, specifically in midfiled positions, that the experts tried to identify a more multifunctional player profile as opposed to the positions occupied by wingers, which have particularly offensive characteristics. Another drawback encountered, in order to ensure the construct validity of the tool, was that the positions of creative and box-to-box midfield share spatial filtering, i.e. many players were rated in both positions, as they performed in the central area of the field. This resulted in many players who scored high as creative midfielders obtaining low scores as box-to-box midfielders, due to the variables of analysis being different. Their market value also skewed the results, since the best creative midfielders (De Bruyne, Maddison, David Silva, Tony Kroos, Odegaard…) had higher market values than the best box-to-box midfielders (Mikel Merino, Saúl Ñíguez, Fred, Ward-Prowse…). In the case of left-backs in La Liga, no significant relationship was found between rating and market value. This was surely due to the fact that several players with low market values performed at a high level during the season (Estupiñán, José Ángel, Fran Gámez, Lucas Olaza, Toño…) and, at the same time, that several players with high market value did not perform at the level expected (Gayà, Jordi Alba, Mendy…).
As for the limitations of the study, on the one hand, the main limitation of the tool revolves around its dependence on the data provider, since the files were coded taking the database that can be downloaded through Wyscout as a reference. This is a minor shortcoming, as the provider could change the way data is exported at any time, which would require modifications to the tool’s code. On the other hand, it is interesting to mention that variables focused on relationship with the ball, which are widely used to assess performance in team sports, should be judged with care when comparing players from different divisions and categories (Sánchez-López et al., 2023a). Although the leagues may have a similar level of play, the contexts of confrontation may be different due to the cultural system. With regard to this idea, it also seems important to point out that it is necessary to consider the selection variables by position and the weighting of these variables when determining which player in the team may be suitable to play as a starter in a match, or which players on the market may be suitable to bring into the club. Regarding this point, the team’s style of play plays a key role in winning (Kong et al., 2022), as well as in the type of actions that are more relevant to good collective performance. In this sense, the selection of variables and weightings presented in this work have been thoroughly validated, attempting to respond to any style of play. For this reason, it is not recommended that very drastic modifications be made, as some validity could be lost in this process.
Sport sciences are continuously working on the application of new methodologies and training systems to improve and maintain the performance of athletes (Pons Alcalá et al., 2020). Consequently, the instrument validated in this study has innumerable possibilities of applicability in the sporting and academic field, among which the following could be highlighted: (1) within the team itself, it is possible to analyse and compare the performance of players occupying the same position, in order to identify which player may be more suitable for a given match, or which player is performing better in that position according to his play; (2) it is also possible to analyse the performance of players from the team itself and players from teams in the league, establishing comparisons and rankings by playing position; (3) another alternative revolves around the assessment of possible recruits on the basis of the performance of the players; (4) it is also possible to assess the evolution of players by comparing their performance longitudinally, e.g. from one season to another, or in two different periods of a league.
As for future prospects for the tool, they depend directly on the future of the supplier, in the knowledge that the scenario that lies ahead is very exciting, as suppliers are increasingly offering a greater quantity of data, of much higher quality.
Conclusions
As conclusions of the study, TOPSTATS shows optimal validity values. It is an instrument capable of rating, classifying and comparing the football competence shown by professional players in their play during the same competition, according to their playing position. To this end, the tool allows for the agile and semi-automatic calculation of an overall performance index obtained from the interaction and weighting of variables contained in the data obtained from the Wyscout provider, which has data coverage in more than 200 competitions.
Therefore, the instrument could be used by professional clubs, sports performance analysis departments and coaches to analyse and compare players, allowing for further optimisation of training and evaluation processes. Similarly, in the scientific field, the instrument could be useful in research that requires the creation of study groups around the competitive performance of players.
Declaration of conflict of interest
The authors declare that there are no potential conflicts of interest with respect to the research, authorship and/or publication of this article.
Funding
The authors received no financial support for the research, authorship and/or publication of this article.
References
[1] Anderson, C. & Sally, D. (2014). The numbers game: why everything you know about soccer is wrong. En Choice Reviews Online. London: Penguin Books.
[2] Araújo, D. (2005). A acçao táctica no desporto. Uma persectiva geral. En O context da decisão. A acção táctica no desporto (Visão e co)
[3] Berrar, D., Lopes, P., Davis, J. & Dubitzky, W. (2019). Guest editorial: special issue on machine learning for soccer. Machine Learning, 108, 1-7. https://doi.org/10.1007/s10994-018-5763-8
[4] Bornn, L., Cervone, D. & Fernandez, J. (2018). Soccer analytics: Unravelling the complexity of “the beautiful game”. Significance, Volume 15, Issue 3, June 2018, Pages 26–29. https://doi.org/10.1111/j.1740-9713.2018.01146.x
[5] Brooks, J., Kerr, M. & Guttag, J. (2016). Developing a data-driven player ranking in soccer using predictive model weights. Proceedings of the ACM SIGKDD International Conference on Knowledge Discovery and Data Mining. https://doi.org/10.1145/2939672.2939695
[6] Carvajal, A., Centeno, C., Watson, R., Martínez, M. & Sanz Rubiales, Á. (2011). ¿Cómo validar un instrumento de medida de la salud? Anales del Sistema Sanitario de Navarra, 34(1), 63-72. https://doi.org/10.4321/s1137-66272011000100007
[7] Castellano, J. & Clemente, F. M. (2020). How much does ball possession influence match performance? Integrating physical and tactical data. En Barça Innovation Hub (Ed.), Football Analytics: Now and Beyond (pp. 94-109). FC Barcelona.
[8] Castellano, J. & Echeazarra, I. (2019). Network-based centrality measures and physical demands in football regarding player position: Is there a connection? A preliminary study. Journal of Sports Sciences, 37(23). https://doi.org/10.1080/02640414.2019.1589919
[9] Cohen, J. (1988). Statistical power analysis for the behavioral sciences. New York: Routledge. https://doi.org/10.4324/9780203771587
[10] Coutinho, D., Gonçalves, B., Santos, S., Travassos, B., Folgado, H. & Sampaio, J. (2022). Exploring how limiting the number of ball touches during small-sided games affects youth football players’ performance across different age groups. International Journal of Sports Science and Coaching, 17(3), 545-557. https://doi.org/10.1177/17479541211037001
[11] Cronbach, L. J. & Meehl, P. E. (1955). Construct validity in psychological tests. Psychological Bulletin. 52(4), 281–302. https://doi.org/10.1037/h0040957
[12] Dellal, A., Chamari, K., Wong, D. P., Ahmaidi, S., Keller, D., Barros, R., Bisciotti, G. N. & Carling, C. (2011). Comparison of physical and technical performance in European soccer match-play: Fa Premier League and La Liga. European Journal of Sport Science, 11(1), 51-59. https://doi.org/10.1080/17461391.2010.481334
[13] Dellal, A., Wong, D. P., Moalla, W. & Chamari, K. (2010). Physical and technical activity of soccer players in the French first league- with special reference to their playing position. International SportMed Journal, 11(2), 278-290.
[14] Díez, A., Lozano, D., Arjol-Serrano, J. L., Mainer-Pardos, E., Castillo, D., Torrontegui-Duarte, M., Nobari, H., Jaén-Carrillo, D. & Lampre, M. (2021). Influence of contextual factors on physical demands and technical-tactical actions regarding playing position in professional soccer players. BMC Sports Science, Medicine and Rehabilitation, 13(1). https://doi.org/10.1186/s13102-021-00386-x
[15] Duch, J., Waitzman, J. S. & Nunes Amaral, L. A. (2010). Quantifying the performance of individual players in a team activity. PLoS ONE, 5(6), 1-7. https://doi.org/10.1371/journal.pone.0010937
[16] Errekagorri, I., Castellano, J. & Echeazarra, I. (2020). Analysis of the ball possession in youth soccer in relation to situational variables: Case study. Cuadernos de Psicología del Deporte, 20(2). https://doi.org/10.6018/CPD.370261
[17] Firiteanu Vasile, N. (2013). The technical study for different game positions in the 2nd League. Ovidius University Annals, Series Physical Education & Sport/Science, Movement & Health, XIII(2), 795-802.
[18] Garganta, J. & Gréhaigne, J. F. (1999). Abordagem sistêmica do jogo de futebol: Moda ou necessidade? Revista Movimento: Universidad Federal de Rio Grande du Sol: Brasil., 5(10), 40-50. https://doi.org/10.22456/1982-8918.2457
[19] Gréhaigne, J.-F., Bouthier, D. & David, B. (1997). Dynamic-system analysis of opponent relationships in collective actions in soccer. Journal of Sports Sciences. https://doi.org/10.1080/026404197367416
[20] Hernández Moreno, J. (1995). La diversidad de prácticas. Análisis de la estructura de los deportes para su aplicación a la iniciación deportiva. En D. Blázquez (Ed.) La iniciación deportiva y el deporte escolar (pp. 287-310). Barcelona: INDE.
[21] Hernández, R., Fernández, C. & Baptista, P. (2010). Metodología de la Investigación. México DF: McGraw Hill.
[22] Hughes, M., Caudrelier, T., James, N., Redwood-Brown, A., Donnelly, I., Kirkbride, A. & Duschesne, C. (2012). Moneyball and soccer - An analysis of the key performance indicators of elite male soccer players by position. Journal of Human Sport and Exercise, 7(2), 402-414. https://doi.org/10.4100/jhse.2012.72.06
[23] Izzo, R., Rossini, U., Raiola, G., Cejudo Palomo, A. & Hosseini Varde’I, C. (2020). Insurgence of fatigue and its implications in the selection and accuracy of passes in football. A case study. Journal of Physical Education and Sport, 20(4), 1996-2002. https://doi.org/10.7752/jpes.2020.04269
[24] Konefał, M., Chmura, P., Zajac, T., Chmura, J., Kowalczuk, E. & Andrzejewski, M. (2019). A New Approach to the Analysis of Pitch-Positions in Professional Soccer. Journal of Human Kinetics, 66, 143-153. https://doi.org/10.2478/hukin-2018-0067
[25] Kong, L., Zhang, T., Zhou, C., Gómez, M.-A., Hu, Y. & Zhang, S. (2022). The evaluation of playing styles integrating with contextual variables in professional soccer. Frontiers in Psychology, 13, 1002566. https://doi.org/10.3389/fpsyg.2022.1002566
[26] Landis, J. R. y Koch, G. G. (1977). The Measurement of Observer Agreement for Categorical Data. Biometrics, 33, 159-174. https://doi.org/10.2307/2529310
[27] Lasierra, G. (1993). Análisis de la interacción motriz en los deportes de equipo. Aplicación de los universales ludomotores al balonmano. Apunts Educación Física y Deportes, 32, 37-53.
[28] León, O. G. & Montero, I. (2007). A guide for naming research studies in Psychology. International Journal of Clinical and Health Psychology, 7(3), 847-862.
[29] Liu, H., Hopkins, W., Gómez, M. A. & Molinuevo, J. S. (2013). Inter-operator reliability of live football match statistics from OPTA Sportsdata. International Journal of Performance Analysis in Sport. https://doi.org/10.1080/24748668.2013.11868690
[30] McDowell, I. & Newell, C. (1996). Measuring healh: a guide to rating scales and questionnaires. Oxford: Oxford University Press.
[31] Nieto, S., Castellano, J. & Echeazarra, I. (2022). Description of collective behaviour in football according to the level of competence in representative tasks from positional data: Systematic review. International Journal of Sports Science and Coaching, 17(6), 1553-1566. https://doi.org/10.1177/17479541221088640
[32] Otero-Saborido, F. M., Aguado-Méndez, R. D., Torreblanca-Martínez, V. M. & González-Jurado, J. A. (2021). Technical-tactical performance from data providers: A systematic review in regular football leagues. En Sustainability (Vol. 13, no. 18). https://doi.org/10.3390/su131810167
[33] Pappalardo, L., Cintia, P., Ferragina, P., Massucco, E., Pedreschi, D. & Giannotti, F. (2019a). PlayeRank: Data-driven performance evaluation and player ranking in soccer via a machine learning approach. ACM Transactions on Intelligent Systems and Technology, 10(5), 1-27. https://doi.org/10.1145/3343172
[34] Pappalardo, L., Cintia, P., Rossi, A., Massucco, E., Ferragina, P., Pedreschi, D. & Giannotti, F. (2019b). A public data set of spatio-temporal match events in soccer competitions. Scientific Data, 6(236). https://doi.org/10.1038/s41597-019-0247-7
[35] Parlebas, P. (2001). Juegos, Deporte y Sociedad. Léxico de praxiología motriz. Badalona: Paidotribo.
[36] Parlebas, P. (2018). Une pédagogie des compétences motrices. Acciónmotriz, 20, 89-96. https://dialnet.unirioja.es/servlet/articulo?codigo=6435703
[37] Parlebas, P. (2023). Pasado, presente y futuro de la praxiología motriz. Acciónmotriz, 31, 9-19.
[38] Partovi, F. Y. & Corredoira, R. A. (2002). Quality function deployment for the good of soccer. European Journal of Operational Research. https://doi.org/10.1016/S0377-2217(01)00072-8
[39] Pons Alcalá, E., Martin Garcia, A., Guitart Trench, M., Guerrero Hernández, I., Ramon Tarragó, J., Seirul·lo Vargas, F. & Cos Morera, F. (2020). Entrenamiento en deportes de equipo: el entrenamiento optimizador en el Fútbol Club Barcelona. Apunts Educación Física y Deportes, 141. https://doi.org/10.5672/apunts.2014-0983.es.(2020/4).142.07
[40] Ric, A., Torrents, C., Gonçalves, B., Sampaio, J. & Hristovski, R. (2016). Soft-assembled multilevel dynamics of tactical behaviors in soccer. Frontiers in Psychology, 7(OCT). https://doi.org/10.3389/fpsyg.2016.01513
[41] Sánchez-López, R., Echeazarra, I. & Castellano, J. (2021). Validation of a Football Competence Observation System (FOCOS), Linked to Procedural Tactical Knowledge. Sustainability, 13(12), 6780. https://doi.org/https://doi.org/10.3390/su13126780
[42] Sánchez-López, R., Echeazarra, I. & Castellano, J. (2023a). Comparing semi-professional and amateur game contexts in a Gk+4 vs. 4+Gk via Football Competence (Procedural Tactical Knowledge). Retos, 47, 419-429. https://doi.org/10.47197/retos.v47.94576
[43] Sánchez-López, R., Echeazarra, I. & Castellano, J. (2023b). Validación de un instrumento de codificación para analizar los goles en fútbol (CODITAG). Apunts Educación Física y Deportes, 151, 58-69. https://doi.org/10.5672/apunts.2014-0983.es.(2023/1).151.06
[44] Sánchez-López, R., Echeazarra, I. & Castellano, J. (2023c). Validation of “TesTactico for F7”: A tool to analyse Declarative Tactical Knowledge based on a Football Competence Observation System. Cuadernos de Psicología del Deporte, 23(2), 223-239. https://doi.org/https://doi.org/10.6018/cpd.526421
[45] Thomas, J. R., Nelson, J. & Silversman, S. (2011). Research Methods in Physical Activity. Champaign, IL: Human Kinetics.
[46] Van Lingen, B. (1997). Coaching Soccer. Spring City, PA: Reedswain.
[47] Wiemeyer, J. (2003). Who should play in which position in soccer? Empirical evidence and unconventional modelling. International Journal of Performance Analysis in Sport, 3(1), 1-18. https://doi.org/10.1080/24748668.2003.11868269
[48] Yi, Q., Jia, H., Liu, H. & Gómez, M. Á. (2018). Technical demands of different playing positions in the UEFA Champions League. International Journal of Performance Analysis in Sport, 18(6), 926-937. https://doi.org/10.1080/24748668.2018.1528524
[49] Zeng, Z. & Pan, B. (2021). A Machine Learning Model to Predict Player’s Positions based on Performance. International Conference on Sport Sciences Research and Technology Support, icSPORTS - Proceedings, October, 36-42. https://doi.org/10.5220/0010653300003059
ISSN: 2014-0983
Received: January 11, 2023
Accepted: May 12, 2023
Published: October 1, 2023
Editor: © Generalitat de Catalunya Departament de la Presidència Institut Nacional d’Educació Física de Catalunya (INEFC)
© Copyright Generalitat de Catalunya (INEFC). This article is available from url https://www.revista-apunts.com/. This work is licensed under a Creative Commons Attribution-NonCommercial-NoDerivatives 4.0 International License. The images or other third party material in this article are included in the article’s Creative Commons license, unless indicated otherwise in the credit line; if the material is not included under the Creative Commons license, users will need to obtain permission from the license holder to reproduce the material. To view a copy of this license, visit https://creativecommons.org/licenses/by-nc-nd/4.0/deed.en